Automatic Speech Recognition (ASR)
Copy link
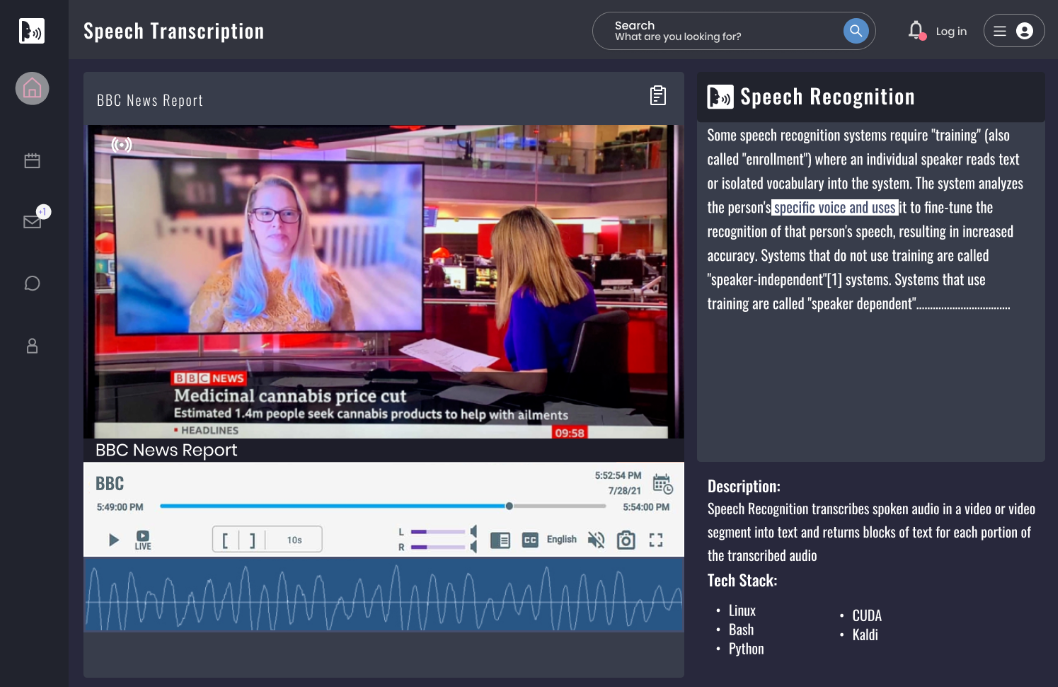
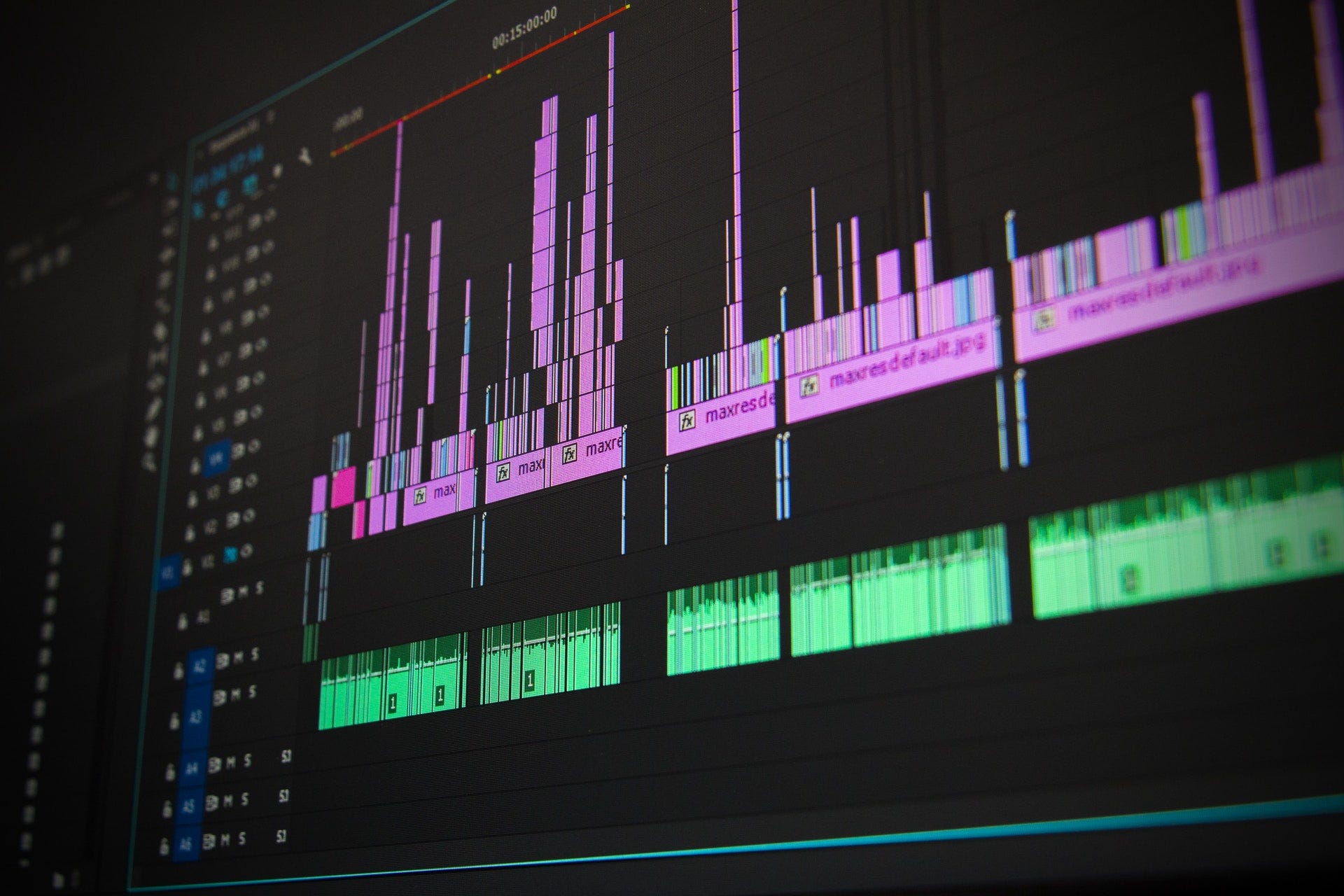
We developed a robust speech recognition engine that transcribes spoken audio in videos and audio recordings into accurate, high-quality text. Our cutting-edge technology empowers businesses and organizations to unlock the valuable insights hidden within their audio and video content.
Tech Stack
Our speech recognition solution is built on a powerful stack of technologies, including:
- Operating System: Linux
- Programming Language: Python
- Libraries and Frameworks: Kaldi, CUDA
- Scripting: Bash
This carefully curated technology stack enables us to deliver a highly accurate and efficient speech transcription service.
Our Use Case
In our specific use case, we have built the speech recognition engine for a media monitoring platform focused on broadcast media (TV, radio, and videos). This engine generates accurate transcriptions of television broadcasts, radio programs, and online videos in multiple languages, including English, German, Dari, Pashto, Hindi, and Urdu.
Real-World Applications
Our speech recognition engine has a wide range of applications in various industries, including:
- Voice Assistants: Enabling natural language interactions and voice commands.
- Voice User Interfaces: Allowing users to control and interact with applications using their voice.
- Call Analytics and Agent Assist: Transcribing customer support calls to improve service quality and agent performance.
- Media Content Search: Indexing and searching audio and video content based on the transcribed text.
- Media Subtitling: Automatically generating subtitles and captions for multimedia content.
Task
Artificial Intelligence